Generative AI vs Traditional AI: Key differences and advantages
Explore the key differences between Generative AI and Traditional AI, understanding their unique strengths, applications, and advantages in modern technology.
Generative AI vs Traditional AI: Key differences and advantages
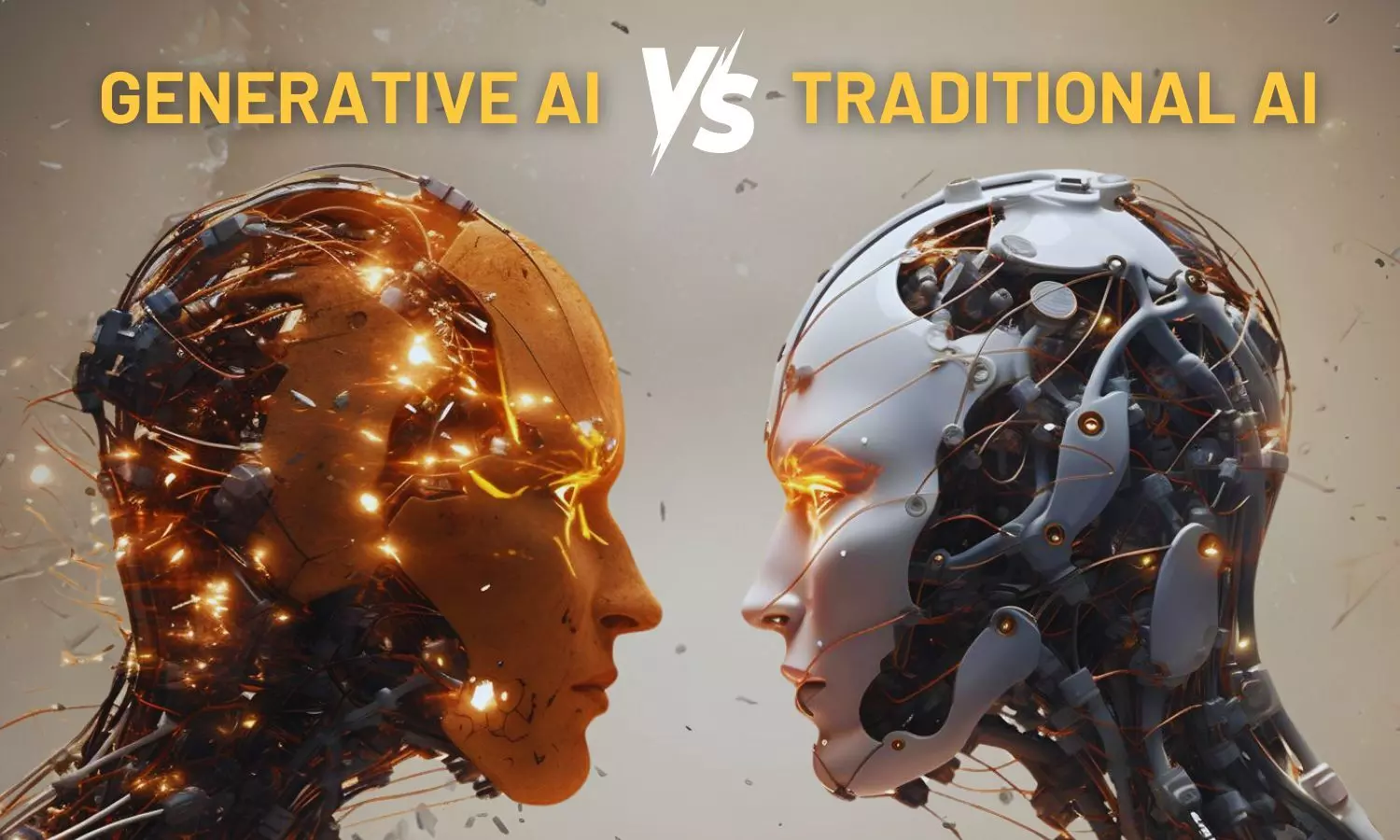
Generative AI vs Traditional AI: How their approaches shape everyday innovations and solutions
The world is changing, it’s like having a machine that can think outside the box and come up with original ideas…
Imagine a world where machines can create stunning artwork, compose music, or even write full-length articles just like humans. This is not science fiction; it’s the reality brought to life by Generative AI. For instance, a recent project saw AI-generated artwork being auctioned for millions, showcasing the creative potential of artificial intelligence. This news story explores the fascinating world of AI, focusing on two main approaches: Generative AI and Traditional AI. Both have unique strengths and applications, shaping how we interact with technology today.
Understanding Traditional AI
Traditional AI, also known as Narrow AI or Weak AI, is designed to perform specific tasks using set rules and algorithms. Unlike General AI, which aims to mimic human intelligence across various activities, Traditional AI excels in one particular area, like spam filtering in emails or playing chess.
Real-world applications
Traditional AI is widely used in everyday life. For example, spam filters in your email service identify unwanted messages based on predefined rules. E-commerce platforms use recommendation systems to suggest products you might like, while virtual assistants like Siri or Google Assistant respond to your voice commands using pre-programmed algorithms.
Understanding Generative AI
Generative AI, on the other hand, focuses on creating new content. Unlike Traditional AI, which sticks to its rules, Generative AI can generate entirely new data, like realistic images, text, or even music. It’s like having a machine that can think outside the box and come up with original ideas.
Applications and examples
Generative AI is making waves across various fields. In art, AI can create original paintings or music compositions. In technology, Generative Adversarial Networks (GANs) can generate lifelike images of people who don’t exist. Language models like GPT-3 can write articles or stories based on a few prompts. This ability to create new content opens up endless possibilities, from virtual reality experiences to drug discovery.
Some key differences between Generative AI and Traditional AI
Approaches: Data-driven vs. Rule-based
Traditional AI relies on rule-based systems, where human experts set specific guidelines for the AI to follow. In contrast, Generative AI is data-driven, learning from vast datasets to create new outputs. It doesn’t follow explicit rules but rather discovers patterns in data to generate something new.
Supervised vs. unsupervised
Traditional AI often uses supervised learning, where the AI is trained on labeled data (for example, images of cats and dogs) to make accurate predictions. Generative AI, however, shines in unsupervised learning, where it finds hidden patterns in data without needing explicit labels. This allows it to generate new content based on the information it has learned.
Discriminative vs. Generative
Traditional AI typically uses discriminative models, which focus on distinguishing between different categories (like classifying an image as a cat or a dog). Generative AI uses generative models that learn the underlying data distribution and can create new samples that resemble the original data, such as generating new, realistic images.
Creativity and adaptability
Traditional AI is excellent at performing specific tasks but lacks creativity and flexibility. Generative AI, however, is designed to be creative and adaptable, capable of generating novel content and adjusting to new situations.
Advantages of Generative AI
Creativity and content generation
Generative AI’s ability to create original content is a major advantage. It can generate new images, texts, music, and even videos, offering endless possibilities for innovation in art, design, advertising, and entertainment.
Handling uncertainty and filling gaps
Generative AI excels at dealing with uncertainty and incomplete data. For example, in medical imaging, Generative AI can create realistic data to improve diagnostic accuracy when real data is scarce.
Applications across industries
Generative AI is opening new doors in various industries. In architecture, it can create virtual models for visualization. In gaming, it generates realistic characters and environments. In virtual reality, it enables immersive experiences by creating interactive content.
Advantages of traditional AI
Efficiency and reliability
Traditional AI is highly efficient at solving specific tasks. It is well-suited for applications that require quick, real-time responses, like industrial automation or autonomous vehicles.
Interpretable results
Traditional AI’s rule-based approach leads to clear and interpretable results. This transparency is crucial in areas like healthcare or finance, where understanding how AI makes decisions is essential.
Proven track record
Traditional AI has a long history of successful applications in industries like robotics and automation, where it has consistently delivered reliable performance.
In summary, Generative AI and Traditional AI offer different benefits depending on the application. Generative AI is powerful in creative and innovative tasks, while Traditional AI excels in efficiency and reliability for specific tasks. Both approaches are shaping the future of AI, offering immense potential for advancements in technology and everyday life.